Assistant Professor of Computer Science Nick Cheney and his collaborators have received the 2023 SIGEVO (Special Interest Group on Genetic and Evolutionary Computation) Impact Award recognizing a paper whose findings are both frequently cited and considered seminal in the field of genetic and evolutionary computation.
Each year SIGEVO, a special interest group of the Association for Computing Machinery (ACM), selects one to three papers presented at the Genetic and Evolutionary Computation Conference (GECCO) held 10 years prior to receive the prestigious award. This year, Cheney along with his colleagues, Robert MacCurdy, Jeff Clune and their advisor at the time, Hod Lipson, received the lone recognition for their 2013 paper, “Unshackling evolution: Evolving soft robots with multiple materials and a powerful generative encoding.”
Inspired by the 1994 work of computer graphics artist and researcher Karl Sims to demonstrate the potential of evolutionary algorithms to produce increasingly more efficient virtual organisms, Cheney and his colleagues sought to understand why, after 20 years of computational advancements, these Darwinian morphologies had not significantly improved in their complexity.
Among the hypotheses explored in their paper was the introduction of soft robots whose structural components were less rigid and, subsequently, less restrictive for design variations. In addition, the paper postulated that a more powerful generative encoding called a compositional pattern-producing network (CPPN) would allow for faster and more diverse evolutionary changes or morphologies.
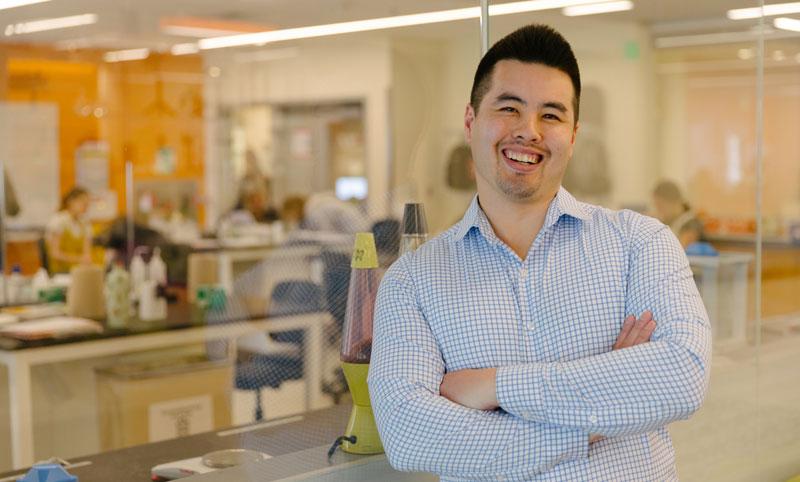
“The idea is to try and take inspiration from biology and build in the type of mechanisms that let us describe complex forms using simple rules and then, iterating them widely, build up forms that are really complex themselves but adapt and mutate easily in an evolutionary system,” said Cheney who was also a received a National Science Foundation CAREER Award this past spring for his groundbreaking research on biologically-inspired neural networks and their implications for machine learning and AI.
By combining an encoding that is based on principles of developmental biology with biologically inspired soft materials that behave similar to muscles and tendons, Cheney and his colleagues were able to create organisms that were not only more life-like in their evolution but also evolved far more rapidly than in previous research.
A video created to accompany the paper in 2013 has garnered hundreds of thousands of views and helps to illustrate the evolutionary cycle of soft robots designed for simple locomotion. Using the fundamental assumption that “faster creatures have more offspring,” the gelatinous virtual creatures walk, squirm, and roll across the screens with varying degrees of efficiency and speed.
“We tried to apply this idea of asking how an evolutionary system would solve the problem of how you design effective soft robots,” said Cheney. “We took the evolutionary approach of providing a bunch of building blocks and then let some evolutionary process try and put them together into what turns out to be these kind of Jello cubes of soft robots that bounce and gallop along the screen.”
Cheney sees the ongoing implications and impact of the paper to be not limited to the field of virtual soft robots. “It is a really unintuitive way of problem-solving using creativity and these bio-inspired algorithms to find solutions,” Cheney said. “I think that having bodies that interact much more richly with their environment allows us to study these cohesive emergent systems between the brain and body.”
That approach to problem-solving translates into active learning experiences for current students taking Cheney’s evolutionary computation course. “It's a nice introduction to computer science in general, but especially the ideas within the field of evolutionary computing and our approach to AI,” said Cheney. “In each generation the steps are simple to explain and, I think intuitive to people, and yet it creates this complexity that relates to designing more adaptable, more efficient, and more robust neural network structures.”