Over the past several decades, theoretical simulations based on quantum-mechanical methods (DFT, etc.) have been crucial in advancing our understanding of vibrational dynamics. However, these calculations are computationally expensive and require long runtimes on even the most advanced high-performance computing (HPC) hardware. Machine learning (ML) methods have recently been used to bridge the gap between the speed of empirical methods with the accuracy of quantum-mechanical methods by learning the complex relationship between atomic positions and potential energy, also known as the potential energy surface (PES). Research in the Ruggiero group focuses on the utilization of neural network potentials (NNPs) to study vibrational dynamics. NNPs attempt to learn the PES from existing databases of quantum-mechanical data or from datasets generated with ab initial molecular dynamics (AIMD). NNPs provide a highly scalable and reusable solution to describe PESs of systems typically not practical for quantum-mechanical approaches, such as proteins and amorphous solids.
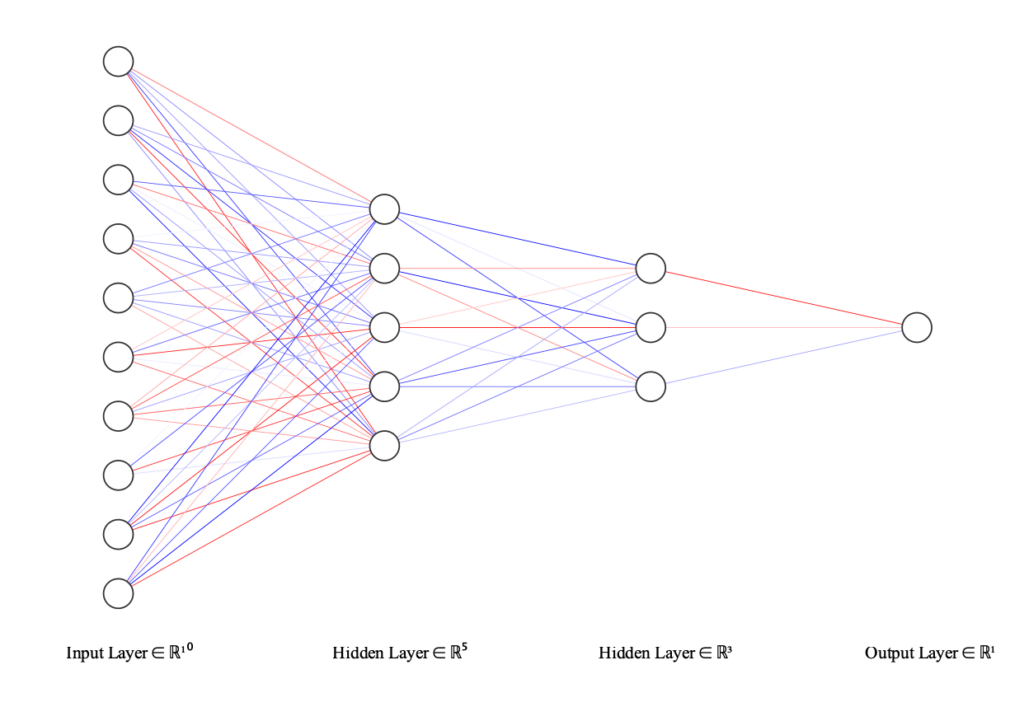