UVM News brings you the University of Vermont’s top stories, showcasing breakthrough research, timely solutions and experts, and news from Vermont’s flagship university.
Featured News
Research For Vermont: Understanding and Mitigating Flood Risk
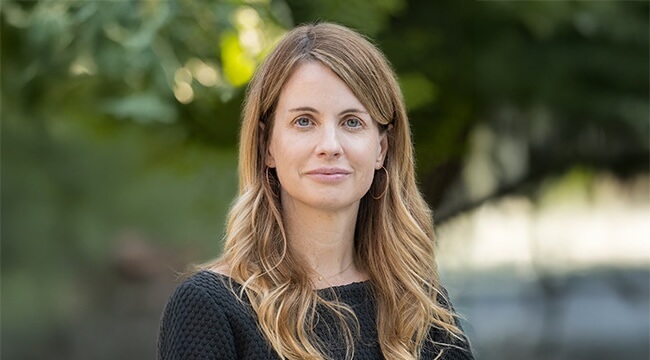
University of Vermont Announces Hiring of Alicia Estey as Vice President for Finance and Administration
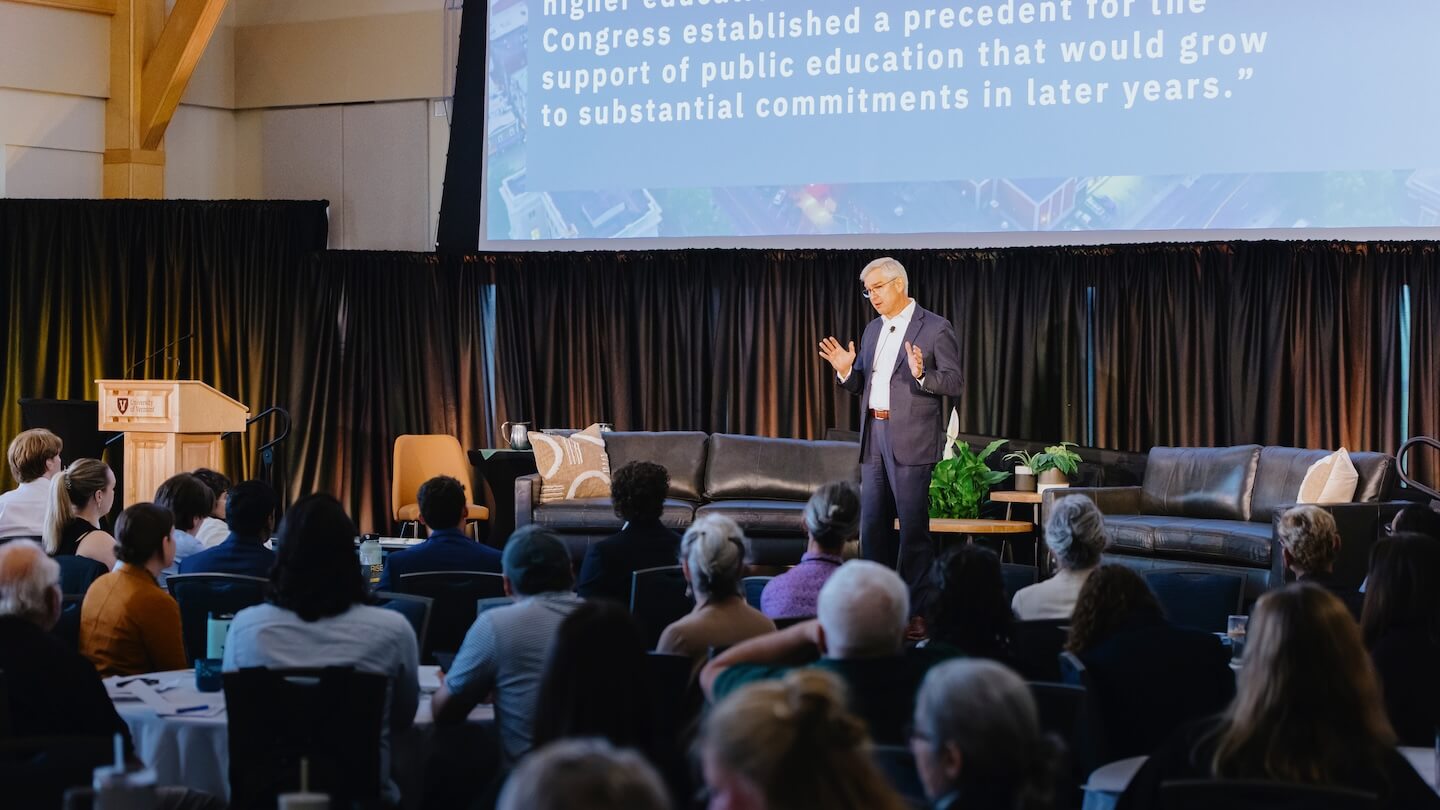
“A Power of People, Projects, and Connections:" UVM’s 2025 RISE Summit Highlights Research as a Public Good
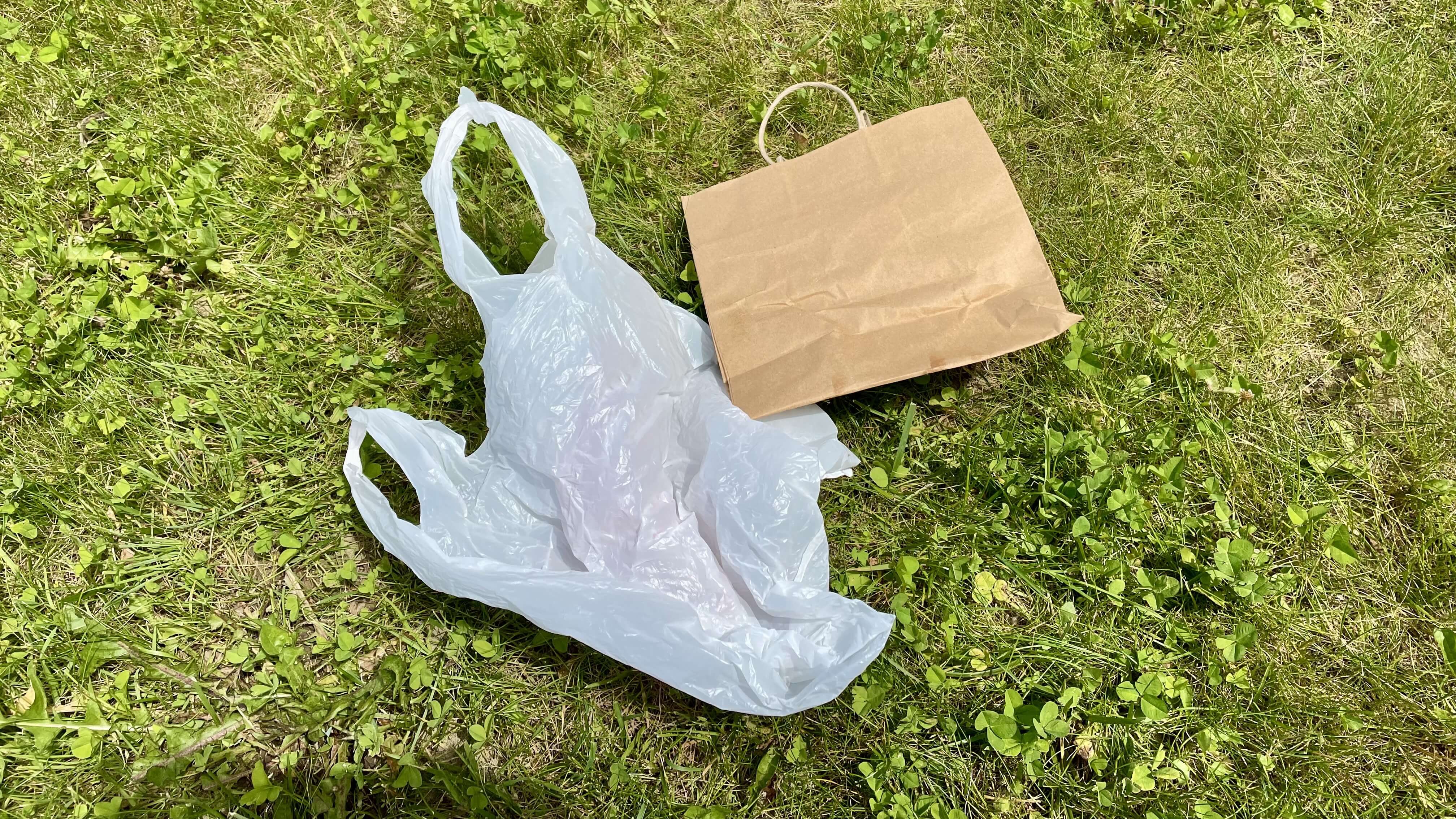
Vermont plastic bag use dropped 91% following ban, researchers find
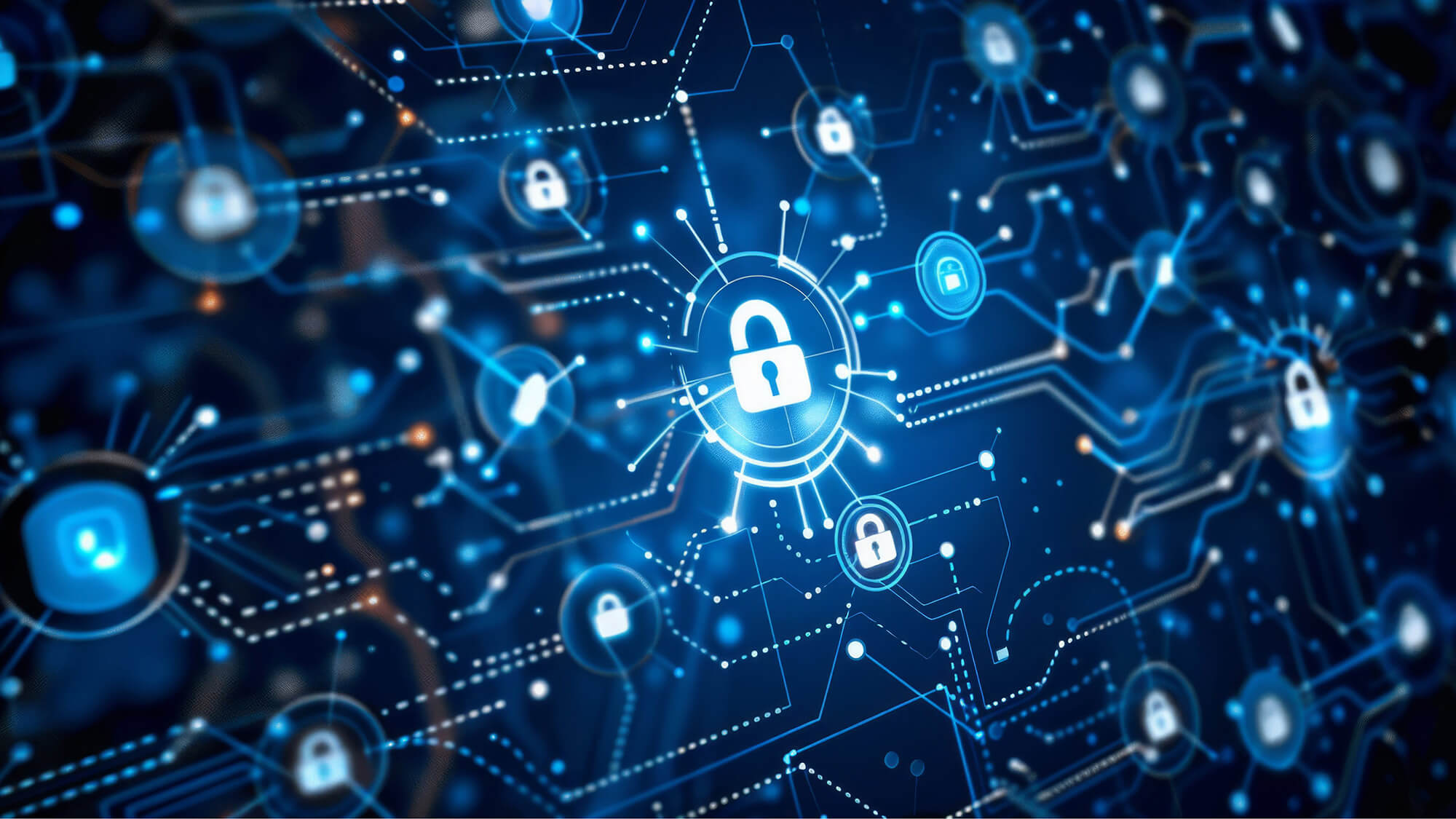
UVM Researchers Get Weird: Bringing Mathematical Rigor to the Science of Hidden Networks