Why Mathematics and Statistics?

Our students and our faculty understand the unique challenges and satisfactions that the careful study of mathematics and statistics offers. Mathematical ideas and results not only represent some of the highest accomplishments of human society, but also are an indispensable tool in an ever-increasing array of disciplines.
The Department of Mathematics and Statistics has a long and proud tradition of excellence in teaching undergraduate students as well as an international reputation for world-class research and mentoring graduate students to a Master's degree or a PhD degree.
Mathematics and Statistics Programs
Student Opportunities
Academic Support
Mathematics and Statistics News
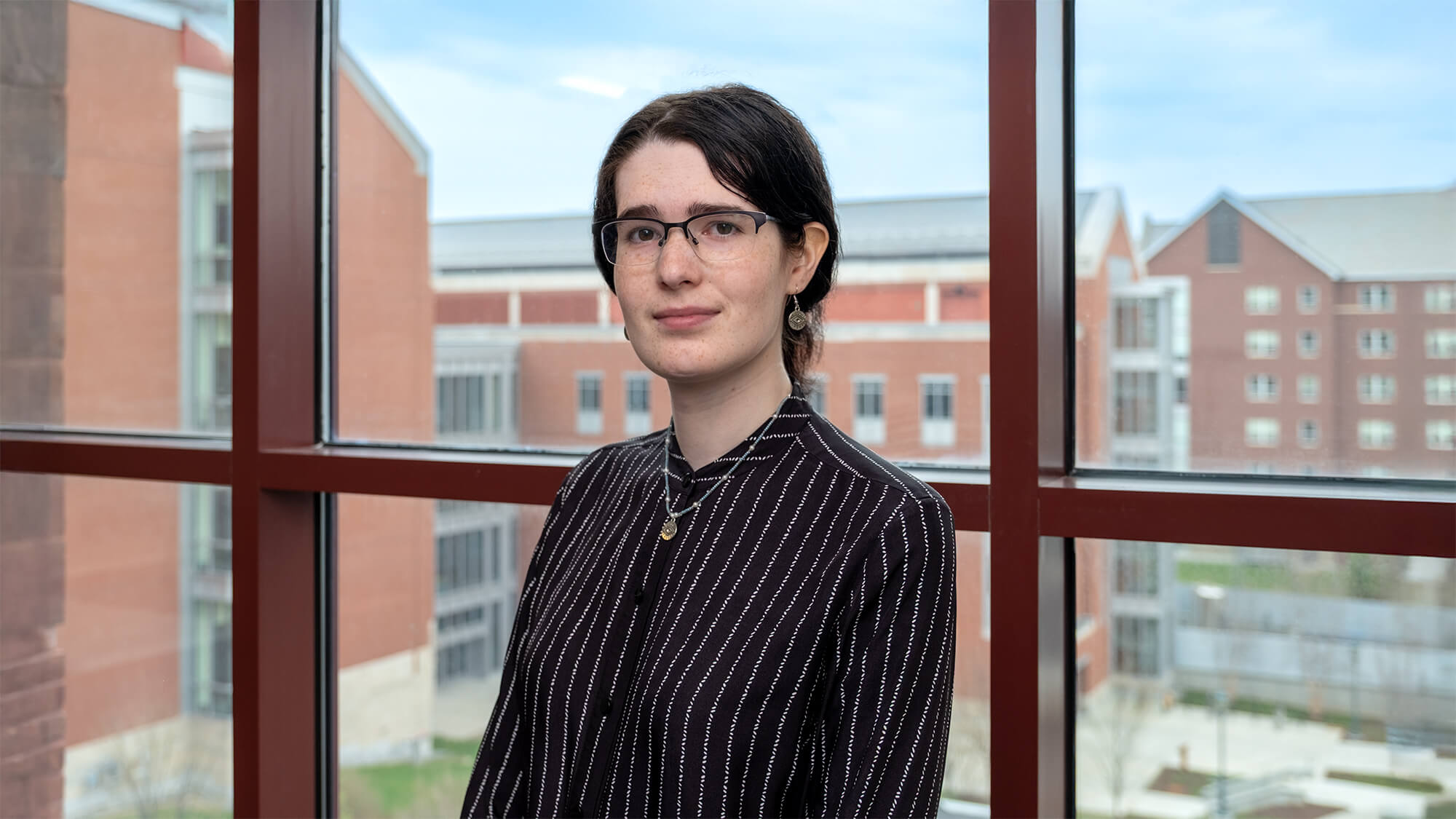
CEMS Senior Spotlight: Erin Silver